Research Article
Ivaldo dos Santos Gomes
Ivaldo dos Santos Gomes
Universidade Estadual do Sudoeste
da Bahia, Campus de Jequié, Departamento de Ciências e Tecnologias, Rua José Moreira Sobrinho s/n,
Jequié – Bahia, 45.206-190, Brazil.
Vinicius Câmara Costa
Vinicius Câmara Costa
Universidade
Estadual de Santa Cruz, Departamento de Ciências Exatas e Tecnológicas, Campus
Soane Nazaré de Andrade, Km 16 - BR-415, Ilhéus - BA, 45662-900, Brazil.
Fábio Alan Carqueija Amorim
Fábio Alan Carqueija Amorim
Universidade
Estadual de Santa Cruz, Departamento de Ciências Exatas e Tecnológicas, Campus
Soane Nazaré de Andrade, Km 16 - BR-415, Ilhéus - BA, 45662-900, Brazil.
Uillian Mozart Ferreira Mata Cerqueira
Uillian Mozart Ferreira Mata Cerqueira
Universidade Estadual do Sudoeste
da Bahia, Campus de Jequié, Departamento de Ciências e Tecnologias, Rua José Moreira Sobrinho s/n,
Jequié – Bahia, 45.206-190, Brazil.
Cleber Galvão Novaes
Cleber Galvão Novaes
Universidade Estadual do Sudoeste
da Bahia, Campus de Jequié, Departamento de Ciências e Tecnologias, Rua José Moreira Sobrinho s/n,
Jequié – Bahia, 45.206-190, Brazil.
Thiago Pereira das Chagas
Thiago Pereira das Chagas
Universidade Estadual do Sudoeste
da Bahia, Campus de Jequié, Departamento de Ciências e Tecnologias, Rua José Moreira Sobrinho s/n,
Jequié – Bahia, 45.206-190, Brazil.
Marcos Almeida Bezerra
Marcos Almeida Bezerra
Corresponding
Author
Universidade Estadual do Sudoeste da Bahia, Campus de Jequié, Departamento de Ciências e Tecnologias, Rua José Moreira Sobrinho s/n, Jequié – Bahia, 45.206-190, Brazil.
E-mail: mbezerra@uesb.edu.br, Tel: +55-73-3528-9600.
Abstract
Industrialized powdered spices are widely used by the
population in food preparation, although they are associated with several
health problems. In this work, six metals (Zn, Fe, Ca, Mg, Na, and K) were
determined in samples of dry industrialized spices that were sprayed and sold
in sachets. Flame atomic absorption (FAAS) and emission (FAES) spectrometry
were used to quantify the metals in the digested samples obtained after the acid
decomposition of the samples in a digester block. The following concentration
ranges were found for the analyzed metals (mg Kg-1): Zn
(<LQ-15.53), Fe (10.82-205.3), Ca (27.45-1842), Mg (114.8-1374), Na
(63739-268188) e K (1560-235864). These values were evaluated using principal
component analysis (PCA) and Kohonen self-organizing maps (KSOMs) techniques.
The multivariate analysis allowed the recognition of grouping trends according
to the spice brands, which suggests the possibility of a feedstock, from which
the flavors are differentiated.
Abstract Keywords
Industrialized
spices, metals, multivariate analysis, PCA, KSOM
1. Introduction
The term “spices” is quite broad and refers to natural
(herbs, seasonings, salts, etc.) or industrialized food additives that add and
enhance specific flavors to foods. It is defined as products obtained from a
mixture of spices and other ingredients, fermented or not, used to add flavor
or aroma to foods and beverages [[i], [ii]]. In addition to
their organoleptic effects, natural spices have physiological benefits, they
are rich in antioxidants, and exert beneficial digestive stimulation through enzymes
responsible for digestion and/or secretion of bile, which plays an important
role in facilitating digestion [[iii], [iv]]. On the other
hand, industrialized spices are strongly discouraged by nutritionists due to
their negative health impacts. They are widely consumed by the population
because they offer practicality, easy access, present low price, and diversity
of flavors in food preparation [[v], [vi]]. The
industrialized powdered spices sold in sachets are made from dehydrated herbs,
salt, coloring agents, condiments, and artificial flavor enhancers such as
monosodium glutamate and disodium inosinate. Their use is heavily criticized
due to the excessive amounts of preservatives, salt, and other additives used
to preserve the product and increase consumer acceptance in terms of flavor.
They can increase the risk of developing heart disease, kidney disease,
diabetes, and even cancer [[vii], [viii]].
The quality of a food depends on several characteristics presented by it, highlighting the diversity and amount of nutrients provided and the absence of harmful contaminating substances [[ix], [x]]. Although the consumption of herbs in nature, such as seasonings in food, brings many health benefits, it is necessary to assess whether nutrient metals and potentially toxic metals are present in safe concentrations for consumption by the population [[xi]-13]. This concern should also be extended to industrialized seasonings due to their high consumption, even knowing all the disadvantages brought by this type of product, as previously discussed.
Modern analytical techniques in food analysis are capable of generating large amounts of data about the samples of interest. However, extracting relevant information from this dataset may not be an easy task when it is done by relying only on human perception. Thus, multivariate analysis techniques for pattern recognition such as principal component analysis (PCA) [14-16] have been widely applied to reveal latent information in the data that cannot be perceived only by performing a visual inspection. In the food area, PCA has been used, for example, to differentiate gelatine sources based on the molecular weights of polypeptides [17], to evaluate the effect of electron beam radiation on ready-to-eat foods [18], to monitor real-time of the coffee roasting process using near-infrared spectroscopy in diffuse reflectance [19], among others.
Kohonen self-organizing maps (KSOMs) are algorithms based on artificial neural networks with the ability to organize complex data into clusters according to their similarities. They adopt a relatively simple and effective approach by reducing the dimension of the data worked, managing to maintain the real representation about to the investigated properties. This method only requests the input data and hyperparameter tuning, the latter being automatically defined by the computing application package, and this is ideal for its application in problems where the patterns are unknown or indeterminate [20]. KSOMs have been applied for, as examples: evaluation of data generated by the determination of metals in tea samples sold in sachets [21], exploration of macro and micronutrient content in samples of soft drinks of different flavors and manufacturers [22], identification of contamination by pathogens in fresh vegetable packages [23], characterization of pine honey from Greece and Turkey after determination of volatile and semi-volatile compounds by mass spectrometry system [24], evaluation of the special and temporal spread of COVID-19 in Brazil according to the number of cases in regions [25], states and cities, study of the use of bees as markers of space-time pollution by lead [26], evaluation of nutrient profiles in samples of cashew nuts [27], performing screening of mineral nutrient content in different parts and two types of passion fruit samples [28], among others.
In the present work, multivariate data analysis techniques
(PCA and KSOMs) were used to explore data generated by analyzing the content of
metals (Zn, Fe, Ca, Mg, Na, and K) by flame atomic absorption (and emission)
spectrometry in samples of industrialized spices sold in sachets. These two
multivariate tools were also compared in terms of efficiency and ability to
recognize patterns of behave about to the analyzed samples.
2.
Materials and methods
2.1 Instrumentation
The determinations of the metals in the digests of the industrialized spice samples were carried out using a Perkin Elmer flame atomic absorption spectrometer (Norwalk, CT, USA) with background correction performed by a deuterium lamp. Except for the elements Na and K that were determined in the emission mode, all the other elements absorption measurements were performed using hollow cathode lamps as radiation source. The wavelengths used for the determination of Ca (422.7 nm), Mg (285.2 nm), Fe (248.3 nm), Zn (213.9 nm), Na (589.0), and K (766.5 nm) were those recommended by the equipment manufacturer. The flame was composed of acetylene gas (2.0 L min-1) and atmospheric air (13.5 L min-1) supplied by a compressor, the sample nebulization flow was 5.0 mL min-1 and the burner height was 13.5 L min-1.
The masses of samples and reagents were determined using a Sartorius analytical balance (Model BL D105, Göttingen, Germany). A Tecnal digester block (Modelo TE 0851, Piracicaba, Brazil) supplied with glass tubes, was used to decompose samples of industrialized seasonings.
2.2 Reagents and solutions
Concentrated nitric acid (Exodus Científica, São
Paulo, Brazil) and 30% hydrogen peroxide (Química Moderna, São Paulo, Brazil)
used in the decomposition of the samples were of analytical grade. To avoid
contamination, the glassware was subjected to a 5% nitric acid bath (vv-1)
for 24 hours, rinsed with ultrapure water, and dried in a dust-free
environment. To obtain ultrapure water, an Elga Purelab Classic system (High
Wycombe, UK) was used. Multielement standard solutions of Ca, Mg, Fe, Na, Zn
and K were obtained by diluting stock solutions (Titrisol, Merck, Darmstadt,
Germany) of each metal in the concentration range from 0.10 to 3.0 mg L-1,
making the calibration solutions. A blank solution was also obtained treating
the reagents used in decomposition in the digester block in the same way as the
sample (but in the absence of the same).
2.3 Sample acquisition
and preparation
The samples of industrialized spices in sachets were purchased in supermarkets in the southwest region of Bahia, Brazil. Thirty-two samples belonging to the four most consumed brands were collected, constituting 8 samples per brand. For each brand, samples belonging to 2 batches were analyzed and the seasonings chosen were those recommended for the preparation of meat, chicken, poultry, vegetables, pasta, salad, beans, and rice.
Each sample was mixed so that there where homogenization, and then they were crushed with the aid of a pestle and mortar, ater they were sieved so that the smallest granules were selected to increase the contact surface, providing more effective digestion. After this step, the samples were weighed on an analytical balance to determine the mass. Approximately 0.3 g of each sample was digested in a digester block, using 2 mL of concentrated HNO3 and 1 mL of H2O2. The digestion process was carried out at 90ºC for approximately 90 minutes. After being cooled, the solutions were made up to 25 mL.
2.4 Data
processing
The data obtained from the determination of metals in
samples of industrialized spices were organized in the form of a matrix
consisting of 96 lines (samples and their triplicates) and 6 columns
(concentration of metals). This data table is presented the in Supplementary
material. Before applying the PCA, the values that constituted the matrix were auto-scaled
to avoid distortions caused by differences in magnitudes both between absolute
values and data variances. The auto-scaling was performed by applying the
following expression:
Where xik is the value to auto-scaled, xk is the mean of the values in a given column, sk is the standard deviation for the column values, and n is the number of objects. Autoscaling was performed using the Microsoft Excel® software. The Statistica® 7.0 program was used to perform the principal components analysis and to generate the score and loading graphs.
Matlab R2016a software (The MathWorks, Co., Natick,
MA, USA) together with a toolbox available at www.cis.hut.fi/projects/somtoolbox
was used for data analysis, generation of graphs, and related figures with
Kohonen's self-organizing maps. The results of the analysis of the spice
samples (concentration of metals in mg Kg-1) were trained and
processed by implementing the codes developed by the algorithms suggested on
the website above.
3. Results and discussion
3.1 Analytical characteristics
The analytical characteristics of the method used to
determine the metals were accessed to prove their suitability in the analysis
of spice samples. Characteristics such as quantification limits, precisions (expressed
as repeatability), sensitivities, and linearity for each metal studied are
presented in Table 1. All values of these figures of merit accessed were
satisfactory and adequate for the methodology used.
Table 1. Analytical
characteristics of the method using FAAS and FAES in the determination of the
metals studied in the spice samples.
Metal |
LOQ (mg/Kg)* |
% RSD** |
Sensitivity (Abs/mg) |
Linearity (R2) |
Zn |
0,15 |
2,9 |
0,373 |
0,9981 |
Fe |
0,17 |
1,8 |
0,0593 |
0,9993 |
Ca |
9,6 |
1,3 |
0,0517 |
0,9982 |
Mg |
0,66 |
3,3 |
0,968 |
0,9980 |
Na |
0,87 |
3,5 |
9752 |
0,9911 |
K |
2,5 |
3,1 |
8952 |
0,9972 |
*Expressed for a sample mass of 0.3 g; **Expressed
in terms of repeatability for 0.5 mg L-1 solutions of the metal and N=10 |
To assess the accuracy of the methodology addiction/recovery
tests and analysis of certified reference material (Apple leaves, NIST 1515) were
carried out. Table 2 presents the results of the analysis of this material. The
paired t-test (95% confidence level) was applied to the two data sets
(certified value and found value) to compare them and verify if they are
statistically similar. The calculated t value (0.9168) was found to be located
in the acceptance region of the Gaussian curve (critical t = 2.57), proving
that the applied methodology has adequate accuracy. Addition/recovery tests were
carried out the by addition of analytes standard solutions in the samples.
Recoveries in the range of 92-101% were obtained showing the method’s accuracy.
Table 2. Results (mean
± standard deviation) of the analysis of certified apple leaf reference
material (N=3).
Metal |
Certified value (mg Kg-1) |
Found value (mg Kg-1) |
Zn |
12,45 ± 0,43 |
11,6 ± 0,6 |
Fe |
82,7 ± 2,6 |
84,3 ± 0,4 |
Ca |
15250 ± 100 |
14587 ± 150 |
Mg |
2710 ± 120 |
3128 ± 181 |
Na |
24,4 ± 2,1 |
25,6 ± 0,8 |
K |
16080 ± 210 |
15287 ± 124 |
3.2 Multivariate
analysis
3.2.1 Principal
component analysis
Principal component analysis was applied to the data
resulting from the analysis and scores (Fig. 1a) and loadings (Fig. 1b) plots
were generated allowing the visualization of the behavior of objects (samples)
and variables (metals concentrations) after the linear transformation of the
data. The first two principal components (PCs) allow the explanation of 58% of
the data variance. The score plot reveals that the analyzed samples tend to be
grouped according to seasoning brands (identified by codes A, B, C, and D)
instead of flavor. This behavior may indicate that manufacturers probably have
a base product from which all flavors are developed and differentiated by
adding small amounts of a mixture of flavorings and flavor enhancers.
Figure 1. (a) Score
plot for data obtained after analysis of samples of industrialized seasonings
sold in sachets; (b) Loadings plot for data obtained after analysis of samples
of industrialized seasonings sold in sachets.
The loadings plot reveals which variables were responsible for separating each group. The concentrations of Fe and Mg were the variables responsible for the formation of the group corresponding to brand D. The concentrations of K and Zn were responsible for the grouping of the samples of brand C. The group formed by the brand B and three samples of the brand B were grouped due to the concentrations of Ca and Na, in addition to the low concentration of Zn and K. Most of the samples of brand A are represented by points that are located near the center of the Cartesian axis, revealing that the concentrations of the variables studied contribute little to differentiate it from the others.
3.2.2 Kohonen
self-organizing maps (SOMs)
SOM is an artificial neural model based on mammals’ brain characteristic of having specific parts that are activated by specific stimuli, being interpreted as a map organized in areas, each area is activated by a specific input that does not activate others areas [[xii]]. Kohonen’s SOM is an artificial neuronal lattice, generally two dimensional, with artificial neurons organized in areas that are automatically trained to be activated by specific characteristics of input data. Each input data is applied to all neurons and the neuron with higher output is more representative with respect to that input characteristics. The lattice is self-organized in the sense that neurons activated by similar inputs are closer and compound a lattice area (or class). Besides that, other areas in the vicinity have low output for the same class of input, evidencing specialized areas.
The routine adopted by Gomes et al. [21] was applied to the data obtained by the
analysis of the samples by FAAS in the implementation of the analysis by KSOM
both in the training stage and the stage of formation of the sample clusters.
Fig. 2b shows the contextual map (8 x 6 dimensions) obtained and its neural
components. Except for two samples (A211 and A111), it is possible to evidence
the formation of four groups related to the different brands of the samples,
regardless of the flavors, as was also observed by the application of PCA. This
map presents the group of neurons that defines the clustering of samples for
various input data due to its ability to reduce dimensionality and eliminate
irrelevant and/or redundant information. Unlike A111, which approaches a group
to which it does not belong, sample A211 forms its own group with
characteristics completely different from the others.
Fig. 2a presents the distance matrix (U matrix) that
shows the formation of groups. In this graph, the proximity of the samples is
based on the color scale, where blue indicates close proximity between the
samples. In it, one can notice the formation of the four groups related to the
brands of industrialized seasonings although the C group presents heterogeneity.
Fig. 2c shows the influence of each determined metal
on the separation of groups according to the seasoning brand. Note that the
group formed by samples of brand D tend to have the highest concentrations of
Fe and Mg. The groups formed by the samples of brand C had the highest
concentrations of K and the group formed by the majority of samples A and B had
high concentrations of Na. Two samples (A211 and B141) formed a separate group
due to their high concentrations of Ca and Na.
Figure 2. a) Distance matrix (U matrix) for the
concentration data generated by the determination of the studied metals in
samples of industrialized seasonings after treatment by KSOM; b) Components of the neural map showing
the neurons responsible for the formation of the groups for the concentration
data generated by the determination of the studied metals in the samples of
industrialized spices after the treatment by KSOM; c) Component maps for the concentration data generated by the
determination of the metals studied in samples of industrialized seasonings
after KSOM treatment.
Although PCA and KSOM revealed very similar latent
information in relation to the analyzed data set, the latter proved to be a
more powerful processing tool, for quick visualization of the formed clusters
and for identifying the variables that influenced the similarity between the
samples. Despite these advantages, KSOMs are still little applied in data
pattern recognition in Analytical Chemistry in relation to PCA, the latter
being much better known and applied.
3.3 Metal
concentrations in spice samples
The concentration averages and dispersion parameters
of the six metals determined in the samples of seasonings in sachets are
presented in Fig. 3. The following general observations can be made: (1) the
concentrations found for Zn were below the limit of quantification in the most
samples mainly for brands A and B; (2) samples of brand D presented the highest
concentrations of elements Zn, Mg and Fe and (3) the highest concentration
ranges occur in relation to Na and K for brand C.
Figure 3. Mean, median
and standard deviation of the concentrations for the elements determined in the
spice samples according to the brands analyzed
4. Conclusions
The determination of the six metals studied in the
samples of industrialized dry spices in sachets and the use of multivariate
analysis techniques allowed to investigate and relate their contents to the
manufacturers who produced them. This behavior may indicate that each
manufacturer uses a base material and differentiates the purpose of the
seasoning by adding small amounts of the ingredients that are responsible for
the flavor. Both principal component analysis and Kohonen's self-organizing
maps allow reaching the same result, that is, the formation of four groups
related to brands. However, KSOMs allow greater precision and security when
grouping the samples, in addition to allowing a quick visualization of the
formed groups and their relationship with the variables responsible for the
formation of the clusters.
Authors’ contributions
Performed the experiments, wrote and revised the manuscript, IS.G. and UM.F.M.C.; Responsible for the statistical treatment, writing and review of the manuscript, V.C.C.C, F.A.C.A. and T.P.C.; Conceived the research project, guided the students in carrying it out and coordinated the writing of the article, M.A.B. and C.G.N.
Acknowledgements
The
authors acknowledge the financial support of the Fundação de Amparo à Pesquisa
do Estado da Bahia (FAPESB), Conselho Nacional de Desenvolvimento Científco e
Tecnológico, and Financiadora de Estudos e Projetos (FINEP).
Funding
Conselho
Nacional de Desenvolvimento Científco e Tecnológico (CNPq, Grant Number
310949/2021-1
Availability of data and materials
All
data will be made available on request according to the journal policy
Conflicts of interest
The authors have no conflicts of interest or competing
interests to declare that are relevant to the content of this article.
References
1.
Srinivasan, K. Spices as influencers of body
metabolism: an overview of three decades of research. Food Res. Int. 2005, 38, 77-86. https://doi.org/10.1016/j.foodres.2004.09.001.a
2.
Brasil, Anvisa RDC N° 276. Ministério da Agricultura e
Pecuária. 2005. Available online:
https://www.gov.br/agricultura/pt-br/assuntos/inspecao/produtos-vegetal/legislacao-1/biblioteca-de-normas-vinhos-e-bebidas/resolucao-rdc-no-276-de-22-de-setembro-de-2005.pdf/view
(accessed on 30 June 2021).
3.
Reinholds, I.; Pugajeva, I.; Bavrins, K.; Kuckovska,
G.; Bartkevics, V. Mycotoxins, pesticides and toxic metals in commercial spices
and herbs. Food Addit. Contam.: B. 2017,
10, 5-14. https://doi.org/10.1080/19393210.2016.1210244.
4.
Platel, K.; Srinivasan, K. Studies on the influence of
dietary spices on food transit time in experimental rats. Nutr. Res. 2001, 21, 1309-1314.
https://doi.org/10.1016/S0271-5317(01)00331-1.
5.
Dossou-Yovo, P.; Tossou, L.T.C.; Sezan, A.; Yelouassi,
R.A.C. Evaluation of the nutritional quality of the most consumed
"cube" broths in South Benin. Int. J. Res. Sci. Innov. Appl. Sci. 2016, 17, 94-99.
http://www.ijias.issr-journals.org
6.
Chen, Z.; Oldewage-Theron, W. Household consumption of
stock cubes and stock powder in the Vaal Triangle of SA. Nutr. Food Sci. 2004,
34, 174. https://doi.org/10.1108/00346650410544873.
7.
Lu, B.; Yan, J.; Yang, X. Effects of sodium depletion
on detection thresholds for salty taste in rats. Physiol. Behav. 2009, 97, 463-469. https://doi.org/10.1016/j.physbeh.2009.03.022.
8.
Li, D.F.; Jia,
D.Y.; Yao, K. Applications and prospects of food additives in complex
seasonings. Chin. Condiment. 2011, 21, 1-4. https://api.semanticscholar.org/CorpusID:101317727.
9.
Qin, G.; Niu, Z.; Xiang, P. Soil heavy metal pollution
and food safety in China: Effects, sources and removing technology. Chemosphere 2021, 267, 129205. https://doi.org/10.1016/j.chemosphere.2020.129205.
10. Biswas, C.;
Soma, S.S.; Hossain, M.S. Assessment of heavy metals in farmed shrimp, Penaeus
monodon sampled from Khulna, Bangladesh: An inimical to food safety
aspects. Heliyon 2021, 7, e06587.
https://doi.org/10.1016/j.heliyon.2021.e06587.
11. Aigberua,
A.O.; Izah, S.C.; Isaac, I.U.
Level and health risk assessment of heavy metals in selected seasonings and
culinary condiments used in Nigeria. Biol. Evid. 2018, 8, 6-20. https://bioscipublisher.com/index.php/be/article/view/3496.
12. Lemos, V.A.;
Santos, L.N.; Bezerra, M.A. Determination of cobalt and manganese in food
seasonings by flame atomic absorption spectrometry after preconcentration with
2-hydroxyacetophenone-functionalized polyurethane foam. J. Food Compos. Anal. 2010, 23, 277-281.
https://doi.org/10.1016/j.jfca.2009.11.004.
13. Yaradua, A.I.;
Alhassan, A.J.; Matazu, K.I.; Nasir, A.; Idi, A.; Usman, I.M.; Kanadi, A.M.
Evaluation of heavy metals in agricultural soils from Katsina state Nigeria.
Sustain. Agric. Food Environ. Res. 2019, 7, 297-313. http://dx.doi.org/10.7770/safer-V0N0-art1555.
14. Bro, R.;
Smilde, A.K. Principal component analysis. Anal. Methods 2014, 6, 2812-2831.
https://doi.org/10.1039/C3AY41907J.
15. Rodionova, O.;
Kucheryavskiy, S.; Pomerantsev, A.; Efficient tools for principal component
analysis of complex data — A tutorial. Chemom. Intell. Lab. Syst. 2021, 213, 104304.
https://doi.org/10.1016/j.chemolab.2021.104304.
16. Cozzolino,
D.; Power, A.; Chapman, J. Interpreting and Reporting Principal Component
Analysis in Food Science Analysis and Beyond. Food Anal. Methods. 2019, 12,
2469. https://doi.org/10.1007/s12161-019-01605-5.
17. Azira, T.N.;
Man, Y.B.C.; Hafidz, R.N.R.; Aina, M.A.; Amim, I. Use of principal component
analysis for differentiation of gelatine sources based on polypeptide molecular
weights. Food Chem. 2014, 151, 286-292.
https://doi.org/10.1016/j.foodchem.2013.11.066.
18. Casla, V.G.;
Conrado, N.R.; Gonzalez, M.E.L.; Arribas, L.V.P.; Diez, L.M.P. Principal
component analysis (PCA) and multiple linear regression (MLR) statistical tools
to evaluate the effect of E-beam irradiation on ready-to-eat food. J. Food
Compos. Anal. 2011, 24, 456-464. https://doi.org/10.1016/j.jfca.2010.11.010.
19. Catelani, T.A.;
Santos, J.R.; Páscoa, R.N.M.J.; Pezza, L.; Pezza, H.R.; Lopes, J.A. Real-time
monitoring of a coffee roasting process with near infrared spectroscopy using
multivariate statistical analysis: A feasibility study. Talanta, 2019, 179, 292-299. https://doi.org/10.1016/j.talanta.2017.11.010.
20. Kohonen, T.
Essentials of the self-organizing map. Neural Networks, 2013, 37, 52-65. https://doi.org/10.1016/j.neunet.2012.09.018.
21. Gomes, D.A.S.;
Alves, J.P.S.; Silva, E.G.P.; Novaes, C.G.; Silva, D.S.; Aguiar, R.M.; Araújo,
S.A.; Santos, A.C.L.; Bezerra, M.A.; Evaluation of metal content in tea samples
commercialized in sachets using multivariate data analysis techniques.
Microchem. J. 2019, 151, 104248.
https://doi.org/10.1016/j.microc.2019.104248.
22. Silva, E.S.;
Silva, E.G.P.; Silva, D.S.; Novaes, C.G.; Bezerra, M.A. Evaluation of macro and
micronutrient elements content from soft drinks using principal component
analysis and Kohonen self-organizing maps. Food Chem. 2019, 273, 9-14. https://doi.org/10.1016/j.foodchem.2018.06.021.
23. Siripatrawan,
U. Self-organizing algorithm for classification of packaged fresh vegetable
potentially contaminated with foodborne pathogens. Sens. Actuators B: Chem. 2008, 128, 435-441. https://doi.org/10.1016/j.snb.2007.06.030
24. Tananaki, C.;
Thrasyvoulou, A.; Giraudel, J.L.; Montury, M. Determination of volatile
characteristics of Greek and Turkish pine honey samples and their
classification by using Kohonen self organising maps. Food Chem. 2007,
101, 1687-1693. https://doi.org/10.1016/j.foodchem.2006.04.042.
25. Galvan, D.;
Effting, L.; Cremasco, H.; Conte-Junior, C.A. The Spread of the COVID-19
Outbreak in Brazil: An Overview by Kohonen Self-Organizing Map Networks.
Medicina 2021, 57, 235-253. https://doi.org/10.3390/medicina57030235
26. Zarić, N.M.; Deljanin, I.; Ilijević, K.; Stanisavljević, L.; Ristić, M.;
Gržetić, I. Honeybees as sentinels of lead pollution: Spatio-temporal
variations and source appointment using stable isotopes and Kohonen self-organizing
maps. Sci Total Environ. 2018, 642,
56-62. https://doi.org/10.1016/j.scitotenv.2018.06.040.
27. Moreira, L.S.; Chagas, B.C.; Pacheco, C.S.V.; Santos, H.M.; Menezes,
L.H.S. Development of procedure for sample preparation of cashew nuts using
mixture design and evaluation of nutrient profiles by Kohonen neural network.
Food Chem. 2019, 273, 136-143. https://doi.org/10.1016/j.foodchem.2018.01.050.
28. Novaes, C.G.;
Romão, I.L.S.; Santos, B.G.; Ribeiro, J.P.; Bezerra, M.A.; Silva, E.G.P.
Screening of Passiflora L. mineral content using principal component analysis
and Kohonen self-organizing maps. Food Chem. 2017, 233, 507-513. https://doi.org/10.1016/j.foodchem.2017.04.111.

This work is licensed under the
Creative Commons Attribution
4.0
License (CC BY-NC 4.0).
Abstract
Industrialized powdered spices are widely used by the
population in food preparation, although they are associated with several
health problems. In this work, six metals (Zn, Fe, Ca, Mg, Na, and K) were
determined in samples of dry industrialized spices that were sprayed and sold
in sachets. Flame atomic absorption (FAAS) and emission (FAES) spectrometry
were used to quantify the metals in the digested samples obtained after the acid
decomposition of the samples in a digester block. The following concentration
ranges were found for the analyzed metals (mg Kg-1): Zn
(<LQ-15.53), Fe (10.82-205.3), Ca (27.45-1842), Mg (114.8-1374), Na
(63739-268188) e K (1560-235864). These values were evaluated using principal
component analysis (PCA) and Kohonen self-organizing maps (KSOMs) techniques.
The multivariate analysis allowed the recognition of grouping trends according
to the spice brands, which suggests the possibility of a feedstock, from which
the flavors are differentiated.
Abstract Keywords
Industrialized
spices, metals, multivariate analysis, PCA, KSOM

This work is licensed under the
Creative Commons Attribution
4.0
License (CC BY-NC 4.0).
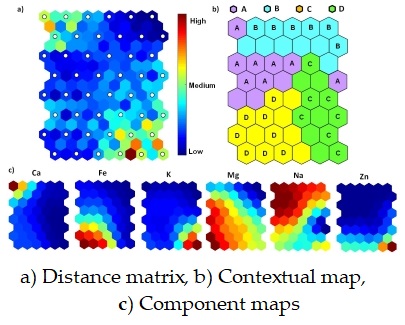

This work is licensed under the
Creative Commons Attribution 4.0
License.(CC BY-NC 4.0).